This guide is designed to help online retailers of every shape, size, and industry to follow ecommerce site search best practices that will increase conversion rates and revenue.
It’s EVERYTHING we know about site search for ecommerce stores.
Read on to learn how to optimize your search experience based on your customers’ shopping behavior, analyze your search reporting, and continuously iterate to deliver hyper-relevant results.
Ready to elevate your site search?
Chapter 1
How much difference can site search really make?
To be blunt, your shoppers who search are simply more valuable than other types of shopper you have.
The average conversion rate of all shoppers is about 1.72%.
However, shoppers that interact with search at least once convert 3.5x – 4.5x more often.
To put it another way, ConversionXL found that site search in some cases made up nearly 40% of a company’s overall revenue while only being used by 10% of the site’s traffic.
ScreenPages did an independent conversion study and found similar results. Less than 10% of shoppers were using search and nearly 40% of overall revenue was coming from those visitors.
We’ve seen some stores with about 50% of their revenue being driven by through their site search.
Whether you’re at the low or high end – site search holds a tremendous amount of promise.
Chapter 2
Is my native platform’s site search enough or do I need more?
First, any site search is better than no site search. So, let’s start off with saying the site search you currently have through your ecommerce platform is better than nothing – it’s a good first start.
However, at the time of this writing, there’s not a single ecommerce platform that is offering an “intelligent” site search solution that will allow you to fully follow ecommerce site search best practices.
They all depend on varying degrees of basic keyword matching.
By comparison, speaking purely of our own customer’s results over basic stock site search solutions, the difference is about 20% in conversion rates, and 15% in average order values.
An intelligent ecommerce site search solution leverages your user-generated behavior with a host of other data points to influence how your site search behaves.
Essentially, we’re comparing a blind system vs. one that is continually optimizing.
A site search that never improves and continues to deliver the same results regardless of past performance vs. one that remembers and adapts.
Chapter 3
Why keyword matching isn’t good enough
All ecommerce platforms offer site search that uses single keyword matching.
For example, when a shopper searches for ‘red nike running shoe’ the search bar will display all the results that have each of those keywords present.
That means every product that has those keywords within its product data, regardless of what it is, will be displayed. The more keywords found in the data, the higher the position in the search results.
At first, that doesn’t sound so bad, but here’s the problem.
The search bar never recognizes the shopper’s intent of ‘shoe’, it’s only driven by keyword density.
So, instead of seeing shoes, which match the shopper’s intent, the shopper will see a mish-mash of shoes and other assorted items (gym bags, shirts, pants, shoe laces, etc).
Any items that contain these words in their searchable fields will display.
If there is any product relevancy, it’s typically by chance.
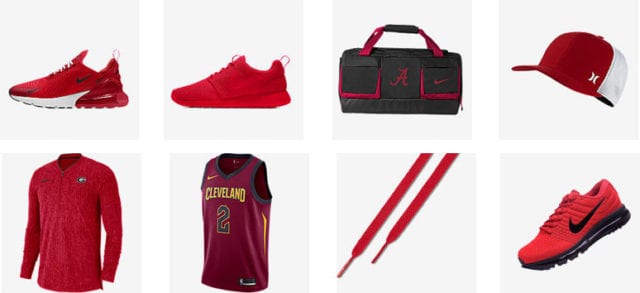
Ecommerce site search best practice:
Using Google Analytics, take your top few on-site search terms and test your store’s relevancy. Are shoppers seeing the products they expect or are there a lot of accessories or other irrelevant items muddying up the results?
Chapter 4
How to optimize your product data for site search
Even the most advanced search solutions in the world are only ever as good as the product data they have to work with.
Improve your data feed structure
Every ecommerce platform has a data feed that powers the product pages and contains all the product data needed to display items. If this feed is rudimentary or missing data, the storefront will reflect that.
Improving the data feed can have a positive impact on product search relevancy, category navigation, product imagery, product descriptions, SEO, merchandising, etc. The data feed is at the core of ecommerce site search best practices, neglecting it is a crippler.
Pro tip: normalize your data
Irregular capitalization and misspellings inside your data can be very aggravating to shoppers.
For example, options of “blue”, “Blue”, “BLUE”, “bleu” should all be relabeled as “Blue”.
While we’re on the topic of colors, consider whether you really need to list various shades of teal, aquamarine, and midnight blue in your filters, or if a single “blue” option will be easier for shoppers to use.
Sizing is another area where consistent naming will dramatically improve the search and navigation experience for shoppers. Even if your range is as simple as XS – XL, keep it as exactly that. Don’t veer into discrepancies between xs, xtra-small, extra small, and so on. Normalize, normalize, normalize.
More tips on this in our blog post on optimizing product data for site search.
Customize what’s searchable
Define what data fields your search bar is actually allowed to search. We recommend that most everyone have these fields set to be searchable:
- Name / Product title
- Sku / Product Code
- Brand / Manufacturer
- Category / Product type
- Any attributes relevant to your catalog: gender, size, color, make/model, author, artist, etc…
As an ecommerce site search best practice, we generally make the following fields not searchable:
- Numeric fields with the exception of measurements
- Price – We of course leave this field filterable, but not searchable
- Description – This one tends to bring a lot of questions, but the description field can be problematic if it’s searchable. It injects a lot of noise that dilutes the relevancy of the product results. For example: A description field could have this line in its copy: “This shirt is great for the beach, be sure to pair it with linen pants.” Now, this shirt has a high probability of showing with any “pant” related search. Of course there are exceptions, but 9 times out of 10, we prefer to leave this field unsearchable.
Chapter 5
How to deliver more relevant product results
First, let’s define product relevancy in the context of ecommerce site search best practices, so we’re both on the same page here.
Simply put, relevancy is all about showing a shopper the products that best match their intent and expectations.
Obviously, easier said than done.
The end result sounds rather simplistic, but the methodologies and algorithms that get you there are far from it.
Product awareness / Natural Language Processing algorithms
A Product Awareness algorithm does two things really well.
- It reads product data in your database and builds an understanding of what each item is.
- It translates each shopper query for its intent – deciphering what is intended to be the product type vs a supporting attribute.
In other words, it can understand that a user searching for “red Nike shoe” wants a shoe, and a user searching for “red Nike shoe laces” wants an accessory for Nike shoes, and delivers the expected results.
This is also often known as semantic search. Semantic search looks at the subtleties in a search query to classify or group products. In just a few milliseconds, it is able to break apart the words in a query to identify which words are product categories, and which are attributes.
This ultimately ensures that shoppers will be taken to the correct set of products, rather than a collection of products that are only loosely related to their query.
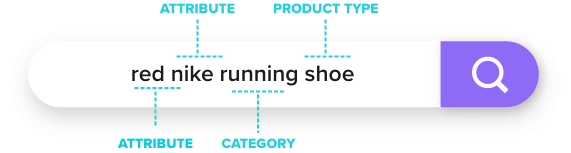
Intelligence / machine learning / AI algorithms
Monitoring, learning, and leveraging the behavior of shoppers is another extremely accurate way to increase the relevancy of results and ensure your site is following ecommerce site search best practices.
While a product awareness engine is able to determine which products are relevant to a query, intelligence goes a step further and ranks products based on how shoppers have engaged with them in the past.
An intelligence layer can leverage valuable data gathered by Google Analytics and other sources in order to “crowd-source” data.
Each time a visitor interacts with a site, searches, clicks a facet/filter, sees products, clicks products, adds products to cart, purchases, etc… data is gleaned that helps to rank products in future searches.
Chapter 6
Types of search queries you’re getting
There’s a lot of nuance behind the psychology of shoppers who use search.
When you understand the “why” of the “how”, you can dramatically improve the shopping experience. It’s important to understand that the buyer’s journey can be anywhere from a few hours to a few months depending on a number of factors.
For example, people shopping for clothes will likely have a much shorter timeline from research to purchase than those shopping for laptops.
In this context, there are 3 basic phases of search visitors.
Exploration – Those in the “exploration” phase are generally only window shopping.
As an example, a shopper on a fashion site might be looking for an outfit that reflects this season’s trends, but they may not know exactly what they’re looking for.
Research – Shoppers in the “research” phase are more specific.
For instance, they might be looking for a pair of shoes, but haven’t necessarily decided which color, brand, or style they want.
Purchase intent – These shoppers know exactly what they want to buy, and how much they are willing to spend.
For example, they might want a pair of white Vans for under $125.
The way shoppers search tells us a lot about what stage of the buying journey they are in.
Search query types
The shopper’s stage in the buying journey, and their intent to purchase, are generally reflected in the following types of search queries. To follow ecommerce site search best practices, your search bar needs to understand this intent, and deliver results accordingly.
Broad Spectrum (Exploration or Research Phase) – Broad spectrum searches are often only one word in length.
A shopper wanting to buy a 4K laptop will search for “laptop”.
Feature (Research Phase) – Feature searches are used to find products that suit a particular need.
A shopper searching for a “4k touchscreen laptop” will do so if they don’t know the names of any laptops that have this feature.
Use Case/Symptom (Research Phase) – Symptom searches are very similar to feature searches. Rather than looking for a specific feature, shoppers are looking for help to solve a particular problem.
A search for “cough medicine” is a good example.
Precise (Purchase Intent Phase) – Precise searches are for exact products or specifications. This shopper has already done their research, and is ready to make a purchase.
A good example would be a search for “512GB MacBook Pro 15”.
Chapter 7
Ecommerce search bar best practices
Get more shoppers to use your search bar. Here’s how.
Actually have a search field
Sounds silly, but topping our list of tips is to actually include a search field, and not hide it.
Hiding the search field is a big no-no.
Stores that only show the search icon or who hide their search bar in a flyout menu are lucky to see minimal shopper engagement with search.
Simply adding the search field can have a significant impact your site search usage.
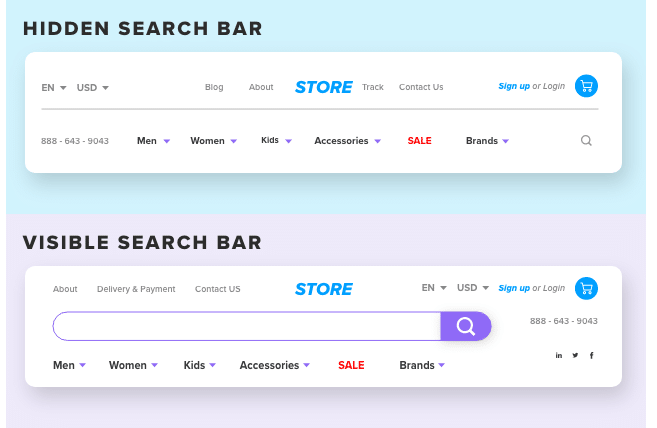
Only have ONE “search” input field
Get rid of any input field that is even remotely close in proximity to your search field.
There’s nothing more frustrating for a shopper than to enter a search and get the “this is not a valid email address” error.
We recommend removing or moving any input field that isn’t the search bar out of view.
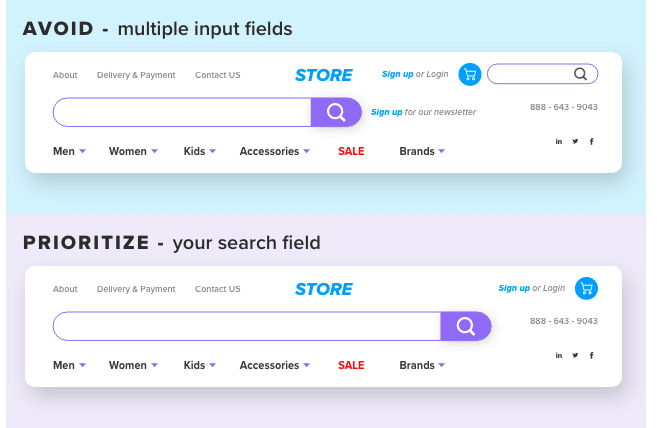
Use a button
Preserving your design and only using an icon with your search field could leave you with a lowly engagement rate.
Adding a button can boost that straight away. Even better, add the obvious “search” text inside the button to boost the engagement rate.
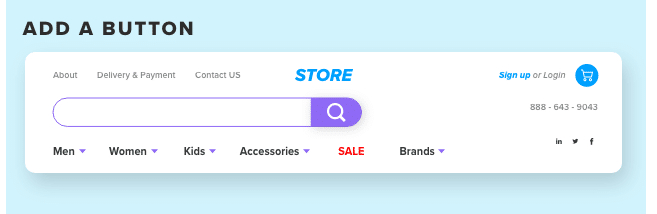
White background and contrasting border
Make your search input field white with a high contrasting border color.
Doing so can lead user engagement rates to soar.
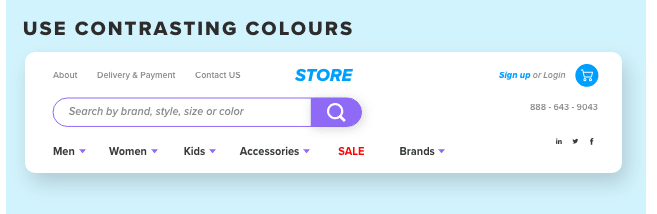
Include contextually relevant and helpful placeholder text
Including placeholder text inside the search input field will boost your engagement rates compared to leaving the search bar empty.
Ecommerce site search best practice: Make the placeholder text instructional and contextually helpful. For example, if you sell media, your placeholder text could say “search by title, publisher, artist, etc…”
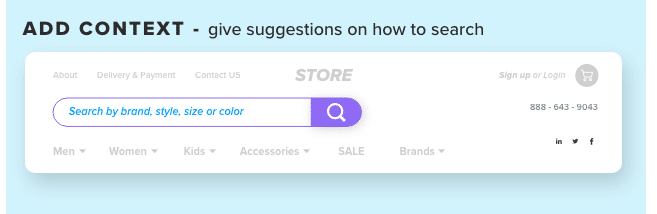
Don’t cram it, add whitespace
Stores that give the search bar an appropriate amount of whitespace see higher engagement rates than stores that “try to save space”.
A good rule of thumb: give the search bar enough room so that a finger could easily select the search input field, on desktop and mobile, without accidentally clicking on something else.
Center the search bar
The common industry convention is to place the search bar on the right side of header navigation. Surprisingly, this isn’t always the best option.
Putting the search bar front, top, and center can boost engagement rates compared to aligning it to the right or left.
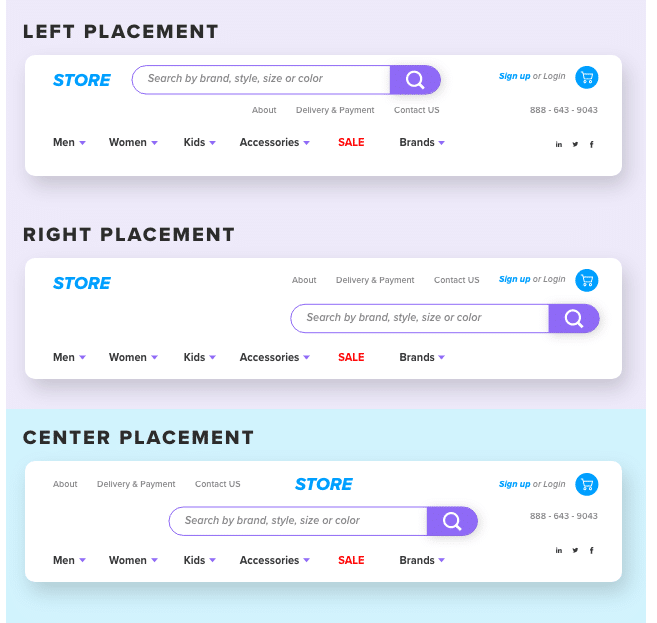
Keep the search bar in the same location
Keep it in the same location, on every page.
Don’t make users hunt for the search bar when they engage with your store. This one is a “common sense” ecommerce site search best practice.
Chapter 8
Autocomplete best practices
Autocomplete should provide two types of suggestions: related searches and product suggestions.
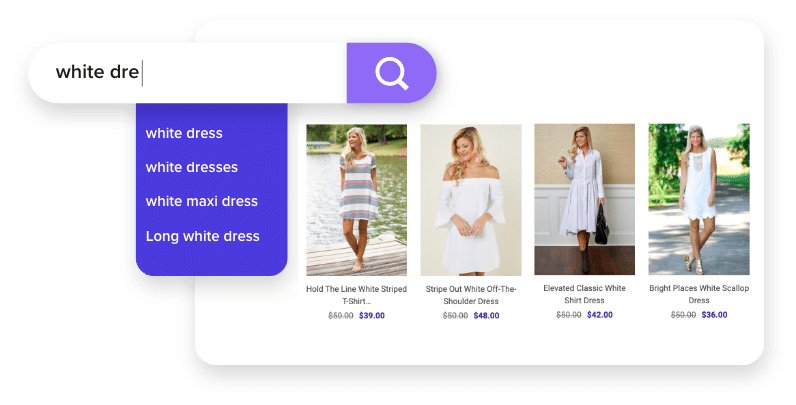
Vary styling for different types of suggestions
Related searches help the user complete their query more rapidly, and will take them to a search results page.
Product suggestions will take the user to a specific product page.
This distinction is important since it sets an expectation for the shopper. If they believe they’re completing a search, they will expect to see a list of products that they can sort and filter on the next page.
If the suggestions look identical, it will create confusion since many users will land on pages that they don’t expect.
We recommend that related searches be text, with a visual emphasis added to the characters the shopper has already typed.
Product suggestions should be placed below the related searches.
Add a border or a colored background to these suggestions for better separation. Also, include product images to convey that clicking will take the user to a product page.
Related searches
Provide typeahead autocomplete suggestions and related search queries for your shoppers.
Why is this an important ecommerce site search best practice? Because shoppers aren’t great spellers. They also usually aren’t sure if they should use their lingo or yours.
Providing related and suggested searches while the user types will help eliminate misspellings, errant searches, and most of all, any confusion your shopper might have.
Product suggestions
When you provide product suggestions directly in the autocomplete, you will see a considerable amount of your traffic navigating directly to those product pages and converting.
So much so, by providing product suggestions you can double engagement and conversion rates from autocomplete.
Limit suggestions so it’s manageable for the user (avoid a long list)
Limit your suggestions to your top few and most relevant, anymore than that will burden the shopper and diminish their experience. If you’re suggesting dozens to hundreds of options then you’re not helping anyone.
Display pricing with product suggestions
Displaying the price with other product data helps shoppers understand that what they are seeing is a specific product which will take them directly to that product page.
Keep the focus inside the search bar and not on the first suggestion
Ensure that your search bar does not select or highlight the first autocomplete suggestion by default. Hitting the enter key should simply search for the query that the shopper has typed into the search field.
Remove redundant suggestions
As a site search best practice, you should do your best to avoid showing over granularization in your suggestions, especially if the suggestions deliver the same results page.
Different spellings of the same word, plural vs. singular, or case sensitivity – bloated suggestions can start to populate for nearly any reason.
Get rid of them. Don’t confuse your shopper into thinking they’ll see different product results when they won’t, and don’t waste your digital real-estate on duplicates.
Support persistent queries
Persistent queries follow the user and retain the query in the search box after the shopper hits “search”.
This is important because the average search visitor enters more than two iterations of their search. Let them modify their search without needing to re-enter their entire search.
Make sure it doesn’t go offscreen
It’s easy to forget, but just because your website is responsive, that doesn’t mean your autocomplete will magically follow your CSS rules. Make sure your autocomplete dropdown doesn’t fly off or get partially hidden at any screen or window size.
Make it accessible
Over 15% of the world’s population and approximately 19% of the US population live with a disability.
Regardless of how few people with disabilities you think might visit your store, ensuring your site search, autocomplete, and navigation are accessible with both the mouse and keyboard will only bring you positive results.
Chapter 9
Ecommerce site search optimizations and best practices
The name of the game is delivering relevant search results. You can make every UI improvement above and use every site search tip below, but if your results aren’t relevant, there’s no saving that.
Here’s how to make your site search smarter and deliver better product results.
Plan for misspellings
Search bars don’t have spell checkers, but that shouldn’t mean the difference of seeing a hundred products vs. zero products.
The ugly truth is, the majority of shoppers will assume your store doesn’t have what they’re looking for before they assume they made a typo. It’s unfortunate, but it’s true.
They think it’s your fault for having a bad search, or that you don’t carry the product they’re looking for. You’ll get the blame before they even consider that it was user error.
If your search bar isn’t optimized to handle simple misspellings, then you are losing a considerable amount of business from your engaged users.
Allow product and SKU code searches
It’s a guarantee, you will have shoppers that try to search products by their SKU or product code. If you’re not returning relevant results from SKU searches, you will miss the sale and lose the shopper.
Bonus points if your search detects and serves relevant results for partial SKU matches.
Create Synonyms and redirects for common zero results
Do searches for “t shirt”, “t-shirt”, and “tshirt” return the same results, or does your search bar get hung up with minor differences?
What about a bigger query difference?
What would your search bar distinguish between “chesterfield” and “couch”? Do you carry “hoodies” or “hooded pullovers”?
You likely have queries your shoppers are using that are returning subpar to no results for products you absolutely do stock.
Dive into your search term report inside GA and know for sure what search terms your onsite shoppers are using.
Support content searches using redirects
You may be surprised to find that some of your most common searches aren’t for products at all, but for content pages.
Whether or not you have a blog, you certainly have pages that don’t have any products. Some of the most common content searches are for “return policy”, “shipping policy”, and “contact us”.
Set up redirects to handle these searches. This alone will help you support the majority of your content searches.
Account for singular and plural keywords
Should “book” and “books” or “dress” and “dresses” be handled the same, or should they display a different set of product results?
We see good reason to treat singular vs plural as the same until there is a specific use case or reason to do otherwise.
Account for special characters (like measurements)
Will your search return the same results for “3 foot cable”, “3’ cable”, and “three foot cable” ?
Setting up synonyms and redirects for every special character variation across all numerical values is virtually impossible.
Ensure your search is smart enough to recognize and account for all these variations.
Make it fast
An obvious but often overlooked ecommerce site search best practice: it should be fast.
Reduce the overall size of your page and make the content your search is delivering as efficient as possible.
1. Use a fast hosting service.
2. Avoid redirects when possible.
3. Minify and concatify your script files to reduce http request.
4. Optimize your shopping cart platform’s configuration.
5. Specify your image dimensions. Optimize your images sizes. Strip out unnecessary image metadata.
6. Use a CDN for your images
7. Enable caching
8. Compress your data with gzip or other compression methods
Chapter 9.A
Merchandise your search results
Merchandising isn’t just for category pages. Boost rules, drag-and-drop controls, banners, badges, and more can be applied to your search result pages to control the order of product display and draw shoppers’ attention to the results you want to highlight.
Yes, an intelligent site search solution will populate the results page with relevant products, but that doesn’t mean you won’t occasionally want to override those results. Maybe you want to promote your new arrivals or offload discounted inventory. Perhaps you have a house brand that should always take precedence.
Merchandising gives you the ability to take control when you need it, and let the technology take over when you don’t. Check out our merchandising guide to learn more!
Level up your ecommerce merchandising
Chapter 9.B
Avoid dead ends
Sometimes shopper’s just search for the wrong things because they don’t know better. It’s an ecommerce site search best practice to always suggest a different route the shopper can take.
- Provide “did you mean” suggestions for semi-relevant and related searches.
- Highlight your popular categories and navigation for browsing.
- If shoppers are searching for common discontinued products or items that you do not carry, create a custom landing page with a relevant alternative.
- Provide an easy method of contact for shoppers to ask questions directly – including a live chat feature is extremely helpful in these scenarios.
You might have to get a little creative depending on your use case, but delivering a shopper to a dead end should be avoided at all cost.
Confirm their search
Oftentimes, people land on no result pages due to incorrect spelling or typographical errors. Displaying their search in large, bold font near the top of the page gives the user an opportunity to see the search they entered and realize their mistake.
Even when the user did not make an error, knowing what they searched for will prevent them from entering the same exact search a second time. This helps them to understand that they need to make a modification to their search in order to find the products they want.
Optimize the “no results found” page
“No results found” doesn’t have to be a dead end. Add links to bestsellers and popular categories on your no results page, or include a guided selling feature to help shoppers get back on track.
If that fails, ensure contact details are prominently displayed so shoppers can get further assistance in tracking down the product they’re looking for – offering more than one communication channel is always a good idea.
Check out our tips on optimizing the no results found page to learn more.
Chapter 10
Learn from your ecommerce site search reporting and insights
Trends, products, and consumers all change rapidly.
Begin checking your data and creating reports on a regular basis to identify your wins and losses – ie. optimization opportunities.
Your shoppers are giving you a goldmine
Every shopper that visits your website leaves data behind. This data should be consistently used to improve and polish relevancy via site search.
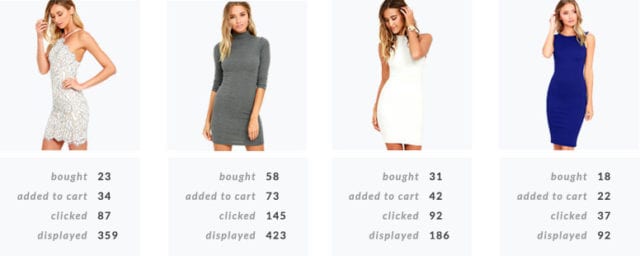
Items viewed, purchased, searched, filters selected in the navigation, discount vs. new product sales performance – it’s all there. Start using it!
Monitor zero results
This is a must-follow ecommerce site search best practice. You will have shoppers that sneak past all your defenses and they will create zero result searches. It’s okay. It’s not the end of the world, but you should never let it persist.
You can minimize zero results by:
- Modifying searchable fields: the odds are good that you already have the shopper’s language within your product data. The problem is, it’s not being utilized because those field values are not searchable.
- Creating custom fields: you will have times when the shopper’s query is nowhere in your data, but it should be. Creating and using the custom fields that your platform makes available is a good way to enhance the product data to capture these types of shopper queries.
- Identifying opportunities for new products: there will be searches for products you don’t carry, but maybe you should. If you have a high volume of searches for a product that isn’t in your catalog, but would make sense to offer, you know you already have the market to begin selling it. Zero results could reveal the low hanging fruit opportunities that shoppers are already looking at you to fulfill.
The list of things you can do when monitoring your zero result searches is hundreds of items long. You can do whatever you like, but the point is, do something. Don’t let the same zero results continue to happen.
Review popular searches with low conversion rates
This is a huge opportunity to increase conversion rates that many retailers are missing out on.
As you review search queries in Google Analytics, take a look at searches that have very low conversion rates, and especially those with zero conversions. Likely, there’s a problem with the results that are loading.
Creating redirects for synonyms with better results or custom-merchandising a landing page are great ways to increase conversions on these pages.
How to find your top searches
Your search terms report can be found in Google Analytics under Behavior > Site Search > Search Terms.
If you’re not seeing any search terms reported, make sure Site Search has been properly enabled within your Google Analytics account and the necessary changes have been made to the tracking code specific to the site search solution currently in use.
From this view, you can see how many times visitors searched for each term, and what they did after completing these searches.
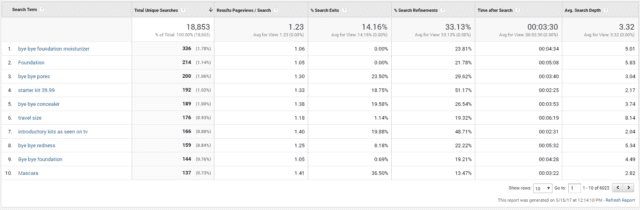
You need to review and type these search terms into your search bar. Are the results relevant?
Next, it’s important to know the bigger picture surrounding the behavior of your search visitors.
In Google Analytics, navigate to Behavior > Site Search > Usage. Here you’ll see a comparison of search visitors vs. non-search visitors.

You’ll be able to see what percentage of your visitors are using search, what their bounce rate is, how much revenue they’re generating, and what percentage of them are converting.
Are these numbers generally higher than non-search visitors?
In most cases, search visitors will have much lower bounce rates, and much higher conversion rates.
Are you failing to see higher conversion rates from search? Focus on fixing search relevancy.
Are you seeing good search conversion rates? Fantastic, now, increase engagement and get even more!
Ready to implement these ecommerce site search best practices? Let’s talk.
With the right insights, tools, and technology, you can transform your site search into an intelligent, user-friendly experience that delivers hyper-relevant results, every time.
If you’re ready to start implementing the site search tips in this guide, but you’re not sure where to start, drop us a line! Our team of ecommerce experts are the best in the business (if we do say so ourselves). They’ll assess your online store to spot the search opportunities you might be missing, and share their insights on how you can elevate your site search game.